Summary: AI networks may become both faster learners and potentially have moral standards by introducing internal reward systems. Recent scientific results indicate a shift in AI. Do we see a new generation of AI?
Artificial intelligence, or AI for short, is all around. Google, Microsoft, IBM, Tesla and Facebook, they are all been doing it for a long time, and this is just the start of it. The Russian president Vladimir Putin recently said that those in the forefront of AI will rule the world, whereas others like Elon Musk and Bill Gates raise concerns regarding the dangers of AI and the creation of super human intelligence.
Where are we heading? Are we in charge, or is the process already beyond control?
One thing is for sure, the speed of development of AI has sky-rocketed since the first attempts were made to mimic human brain learning with simple artificial neural networks several decades ago. Much of the theory behind neural networks has not changed since then, although some algorithmic improvements have come about, like reinforcement learning and convolutional networks, but the real breakthrough of AI in later years has been made possible by brute force, through big data and increased computational power.
Still, the artificial learning algorithms are not as efficient as the natural learning processes of the human brain, yet. Humans are in some respect much more efficient at learning than the computers, although computers may digest much larger quantities of data than us per time unit. We can extract the essence of information (that is, to learn) from only a few repeated examples, whereas a computer may need thousands of input examples in comparison. In some circumstances we may in fact need only a single experience to learn about, for instance, a life threatening danger.
It is no question that the learning algorithms used in AI are computationally heavy and quite inefficient. The AI pioneer Geoffrey Hinton recently expressed he is “deeply suspicious” of the back-propagation step involved in the training of neural networks, and he calls for a new path to AI. Hence, new inspiration is needed to make the algorithms more efficient, and what is then more natural than to turn to the natural neural networks of our own brains for this inspiration?
But faster and more efficient learning does not calm the nerves of those who fear the super human intelligence, on the contrary! How can we be more confident that artificial intelligence will behave according to the moral standards modern and developed societies live by? Also here we should turn to our own brains for inspiration, because after all, humans are capable of thinking and behaving morally, even if the news are filled with counter examples every day. We may still hope that it will be possible to create AI with super human moral standards as well as intelligence!
Geoffrey Hinton is probably right, we need a new path to AI. We need a next generation AI!
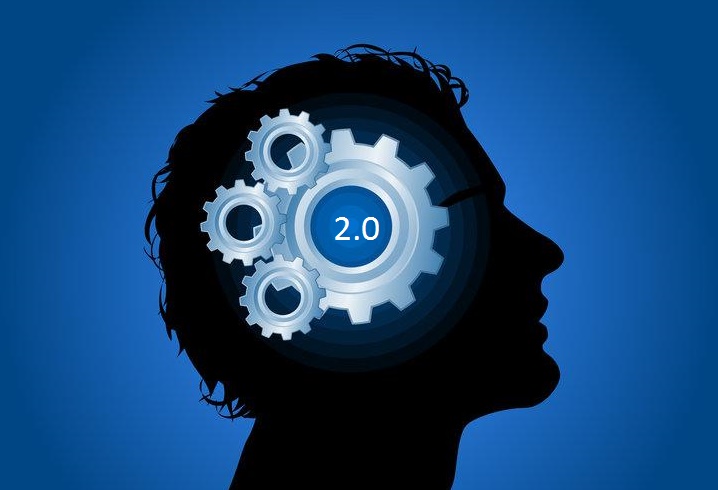
The next generation AI must learn more efficiently and be more human-like in the way it acts according to values and ethical standards set by us.
Three fairly recent scientific findings in AI research and neuroscience may together reveal how next generation AI must be developed.
- The first important result is found within the theory of “information bottlenecks“ for deep learning networks by Naftali Tishby and co-workers at the Hebrew University of Jerusalem.
- The second result is the new curiosity driven learning algorithm developed by Pulkit Agrawal and co-workers at the Berkeley Artificial Intelligence Research Lab.
- And finally, a fresh off the shelf paper by John Henderson and colleagues at the Center for Mind and Brain at the University of California, Davis, shows how visual attention is guided by the internal and subjective evaluation of meaning.
These three results all point, directly or indirectly, to a missing dimension in AI today, namely a top-down control system where higher levels of abstraction actively influence how input signals are filtered and perceived. Today’s AI algorithms are dominantly bottom-up in the way input signals are trained from the bottom and up to learn given output categories. The back propagation step of deep learning networks is in that sense no top-down control system since the adjustments of weights in the network has only one main purpose, to maximize an extrinsic reward function. By extrinsic we mean the outer cumulative reward that the system has been set to maximize during learning.
The fundamental change in AI must come with the shift to applying intrinsic as well as extrinsic reward functions in AI.
Let’s begin with the information bottleneck theory to shed light on this postulate. In a Youtube video Naftali Tishby explains how the information bottleneck theory reveals previously hidden properties of deep learning networks. Deep neural networks have been considered as “black boxes” in the way they are self-learning and difficult to understand from the outside, but the new theory and experiments reveal that learning in a deep network typically has two phases.
- First there is a learning phase were the first layers of the network try to encode virtually everything about the input data, including irrelevant noise and spurious correlations.
- Then there is a compression phase, as deep learning kicks in, where the deeper layers start to compress information into (approximate minimal) sufficient statistics that are as optimal as possible with regard to prediction accuracy for the output categories.
The latter phase may also be considered as a forgetting phase where irrelevant variation is forgotten, retaining only representative and relevant “archetypes” (as Carl Jung would have referred to them).
We may learn a lot about how the human brain works from this, but still, as mentioned above with regard to efficacy of learning, the compression phase appears to kick in much earlier in natural neural networks than in the artificial ones. Humans seem to be better at extracting archetypes. How can this be?
I believe that the information bottleneck properties observed in artificial deep learning networks describe quite closely the learning phases of newborn babies. Newborn babies are, like un-trained AI’s more like tabula rasa in the sense that there are no/few intrinsic higher levels of abstractions prior to the learning phase. Babies also need a lot of observations of its mother’s face, her smell, her sound, before the higher abstraction level of “mother” is learned, just like an AI would.
But here the natural and the artificial networks deviate from one another. The baby may carry the newly learned concept of a mother as an intrinsic prior for categorization as it goes on to learn who is the father, that food satisfies hunger, and so on. As the child develops it builds upon an increasing repertoire of prior assumptions, interests, values and motivations. These priors serve as top-down control mechanisms that help the child to cope with random or irrelevant variation to speed up data compression into higher abstraction levels.
My prediction is therefore that compression into archetypal categories, which has been observed in deep learning networks, kicks in much earlier in networks where learning is both a combination of bottom-up extrinsic learning and top-down intrinsic control. Hence, by including priors into AI, learning may become much faster.
The next question is how priors may be implemented as intrinsic control systems in AI. This is where the second results by Pulkit Agrawal et al. comes in as a very important and fundamental shift in AI research. They aimed at constructing a curiosity-driven deep learning algorithm. The important shift here is to train networks to maximize internal or intrinsic rewards rather than extrinsic rewards, which has been common insofar.
Their approach to building self-learning and curious algorithms is to use the familiar statistical concept of prediction error, a measure of surprise or entropy, as an intrinsic motivation system. Put short, the AI agent is rewarded if it manages to seek novelty, that is, unpredictable situations. The idea is that this reward system will motivate curiosity in AI, and their implementation of an AI agent playing the classic game of Super Mario serves as a proof of concept. Read more about this here.
I believe the researchers at Berkeley are onto something very important in order to understand learning in real brains. As I wrote in an earlier blog post, learning is very much about attention, and attention is according to the salience hypothesis assumed to be drawn towards surprise. So this fits well into the work of Agrawal et al. However, in another blog post I also discussed how attention depends on a mix of extrinsic sensation and intrinsic bias. In a statistical framework we would rephrase this to a mix of input data likelihood and prior beliefs into a posterior probability distribution across possible attention points, and that our point of attention is then sampled randomly from this posterior distribution.
The point here is that prediction error, as a drive for learning, also depends on the internal biases.
These biases are the interests, values, and emotions we all possess that guide our attention, not only towards novelty, but towards novelty within a context that we find interesting and relevant and meaningful.
You and I will most likely have different attention points given the same sensory input due to our different interests and values. These biases actually influence how we perceive the world!
My good friend and colleague, the psychologist Dr. Helge Brovold at the National Centre for Science Recruitment in Trondheim, Norway, states this nicely:
“We don’t observe the world as IT IS. We observe the world as WE ARE”
This has now been confirmed in a recent study by Henderson et al. at the Center for Mind and Brain at the University of California, Davis. They show in experiments that visual attention indeed is drawn towards meaning rather than surprise or novelty alone. This is contrary to the salience hypothesis, which has been the dominant view in later years, according to Henderson. Human attention is thus guided by top-down intrinsic bias, an inner motivation guided by meaning, interest, values or feelings.
As Agrawal and his colleagues implemented their intrinsic prediction-error (or entropy) driven learning algorithm for the Super Mario playing agent, they encountered exactly the problem that some sort of top-down bias was needed to avoid the agent to get stuck in a situation facing purely random (and hence unpredictable) noise. Noise is kind of irrelevant novelty and should not attract curiosity and attention. To guide the algorithm away from noise they had to define what was relevant for the learning agent, and they defined as relevant the part of the environment which has the potential to affect the learning agent directly. In our context we can translate this as the relevant or meaningful side of the environment. However, this is only one way to define relevance! It could just as well be moral standards acting as intrinsic motivation for the learning agent.
At this point we may now argue that the inclusion of top-down intrinsic bias in addition to extrinsic reward systems in deep learning may both speed up the learning process as well as open up for AI guided by moral an ethics. Strong ethical prior beliefs may be forced upon the learning network affecting the learning algorithm to compress data fixed around given moral standards.
In my opinion, this is the direction AI must move.
But… there is no such thing as a free lunch…
The introduction of intrinsic motivation and bias comes with a cost. A cost we all know from our own lives. Biases make us subjective.
The more top-down priors are used and the stronger they are, the more biased learning will be. In the extreme case of maximal bias, sensory input will provide no additional learning effect. The agent will be totally stuck in its intrinsic prejudices. I guess we all know examples of people who stubbornly stick to their beliefs despite hard and contradictory, empirical evidence.
However, the fact that human perception in this way tends to be biased by prior beliefs is, on the other hand, an indication that this is indeed how natural learning networks learn…, or don’t learn…
Permalink
can AI be implemented in a more complex surgery ?
Permalink
Hello. Thanks for making this article and your blog so accessible. I’m researching consciousness and AI for a science fiction story. I don’t understand the phrase “moral an ethics.” Perhaps it’s a typo, or some technical language I’m not familiar with. Could you please clarify? Thank you.
Permalink
Dear Kathy. Thank you for your interest in this blog post! Very interesting to hear that you are working on a fiction story, I would like to hear some time how it turned out 🙂 AI is certainly bringing possibilities for both good use and mis-use to our future. The phrase you are asking about is just a typo. It should say «moral and ethics». I will fix that, thank you 🙂 Good luck on your work!
Permalink
Thank you. If I credit you, may I quote this article in a chapter heading? My story includes AIs that don’t have bodies because they’re built into the facilities they serve, such as a space base or a ship. They’re made with organic components because of my (admittedly layperson) bias that synthetic hardware will never compete with organic brains, at least within the timeline of the story. But the AIs have a sense of embodiment, robust personalities, empathy, and emotions, and they wrestle with moral and ethical dilemmas.
Permalink
Sounds like there’s room for plenty of ethical dilemmas in such a setting, indeed. You are very welcome to credit my blog post!
Permalink
Thank you so much.
Permalink
This is very interesting, Thank you for sharing your article. I really appreciate your efforts and I will be waiting for your further post thanks once again
Permalink
Thank you, zeke! I’m glad to hear! I will soon release the fourth blog post on human and artificial creativity. I hope you will also find that one interesting.